The Role of GPT in Automated Document Classification
Discover GPT's role in document classification: A key to efficiently managing vast data volumes with precision and scalability.
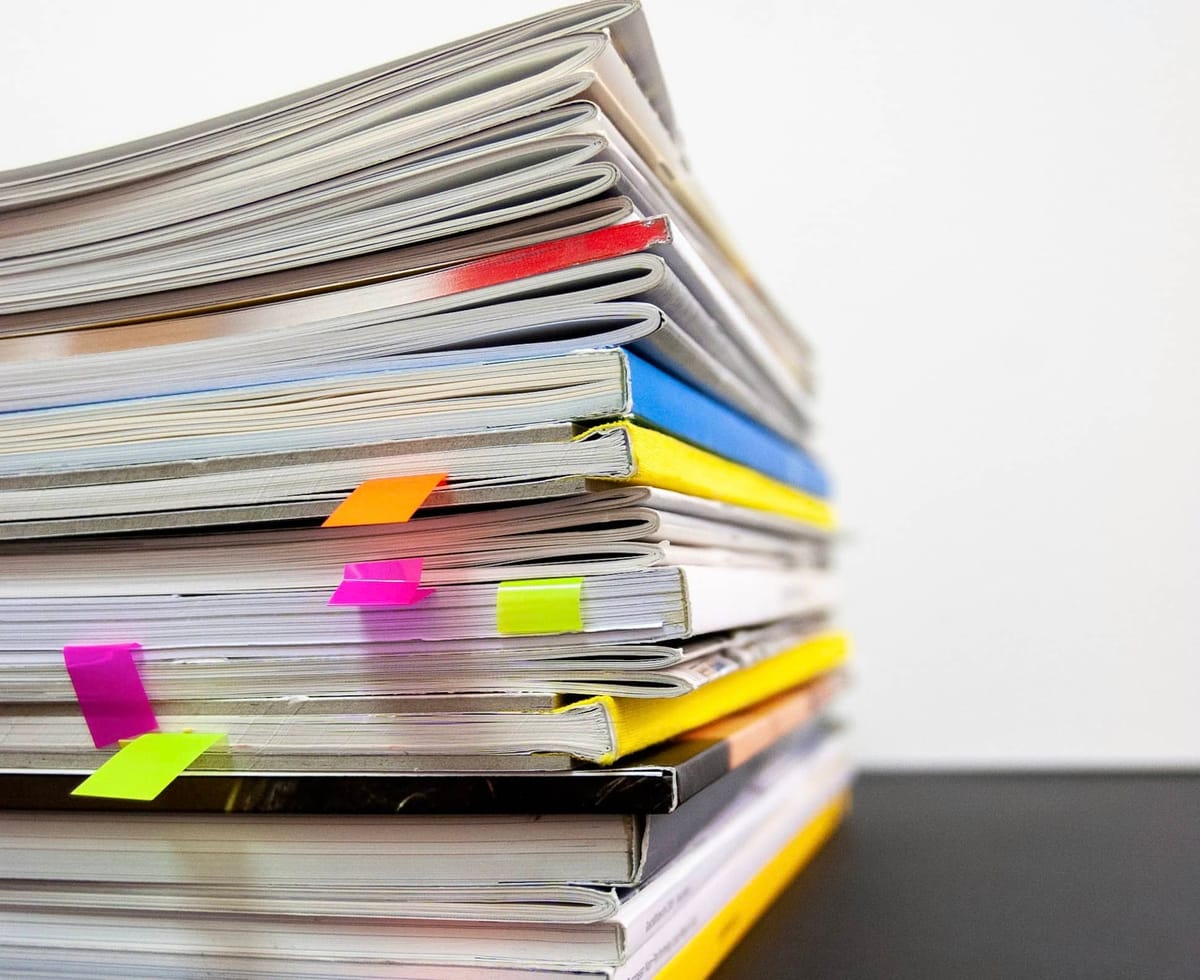
In this digital era, it is quite easy to obtain data with a single click. But imagine having hundreds of terabytes of data but not knowing what you have or not being able to quickly search the intended information in microseconds. This much data will take a lot of time to sort through and to go through it each time you require a piece of information, is a waste of time and resources in this fast-paced world. So, what is the solution to this problem? A simple technological tool is to deploy document classification techniques.
This article will provide insight into the world of document classification, its types, the challenges faced, and the advantages of the currently trending GPT method for document classification.
What is Document Classification?
In simple technical terms, document classification is the process of assigning documents to various categories or classes by distinguishing their traits such as the information that is instilled in them. The categories can vary depending on the type of dataset one has. The use is not limited to areas such as library science, computer science, or information science. The classification can be made for text, videos, images, music, etc. and each type of document requires specialized algorithms to get a perfect classification.
Document Classification Examples and Use Cases
Businesses these days use document classification to extract insights, automate processes, and enhance user experiences. Let's explore some compelling examples and use cases where document classification technologies have proven to be invaluable:
Enhancing Language Detection Capabilities
Language detection tools serve as versatile assets with diverse applications in document classification. By accurately identifying the language of textual data, these classifiers enable companies to route inquiries to appropriate teams, ensuring seamless communication in customers' preferred languages. Moreover, language detection simplifies data management for global teams by organizing documents based on language, facilitating collaboration, and optimizing information retrieval processes.
Trend Analysis from Customer Feedback
Unveiling actionable insights from the deluge of customer feedback is a daunting task that AI text classification tools excel at. By employing machine learning models, businesses can effortlessly identify trends and patterns within product reviews, NPS ratings, and survey responses. This automated analysis empowers organizations to understand customer sentiment, preferences, and pain points, enabling data-driven decisions that drive enhanced customer satisfaction and business growth.
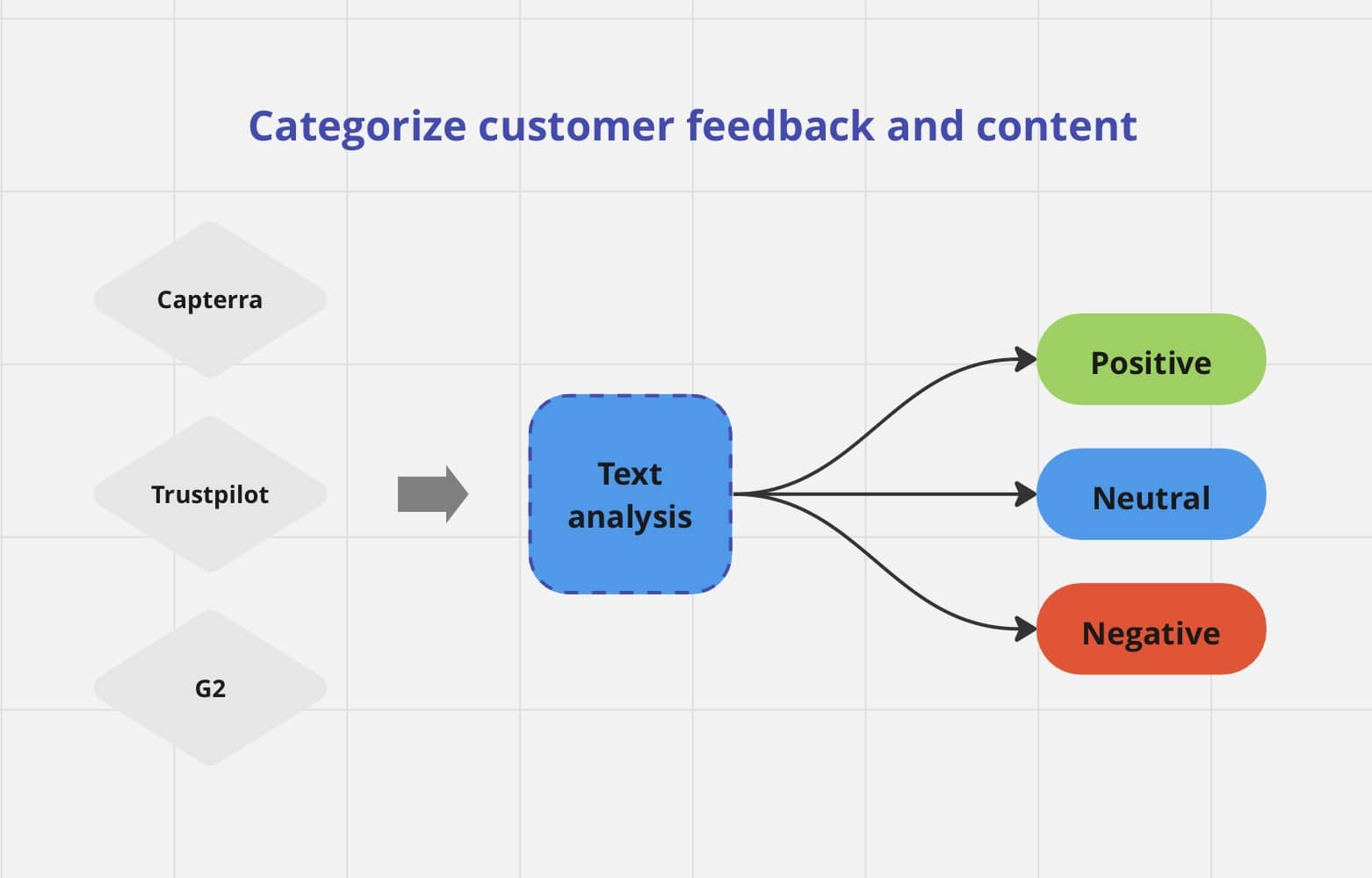
Sentiment Analysis for Enhanced Insights
Sentiment analysis algorithms offer a powerful mechanism for deciphering the emotional nuances of textual content. By determining whether a piece of text conveys positive, negative, or neutral sentiments towards a specific topic or entity, these algorithms provide valuable insights into brand perception, customer satisfaction, and public opinion. Using contextual nuances and language patterns, sentiment analysis tools enable individuals and businesses to gain profound insights into customer sentiments and overall perceptions.
Automated Content Moderation
AI-driven content moderation tools play a pivotal role in upholding online guidelines and policies by leveraging Natural Language Processing (NLP) algorithms. Sentiment analysis techniques enable the classification of user-generated content based on emotions and tones, while entity recognition tools extract valuable insights such as brand mentions and geographical distribution of reviews. By automating content moderation processes, businesses can maintain a safe online environment while gaining valuable demographic data for targeted marketing strategies.
Document Classification Types
As we have discussed, document classification is a crucial task in information management, which enables organizations to organize, retrieve, and analyze large volumes of documents effectively. Two fundamental approaches to document classification include manual and automated methods.
Manual Document Classification involves human operators categorizing documents into predefined classes or categories based on their content, characteristics, or other relevant factors. In this approach, individuals review each document and make classification decisions based on their judgment, expertise, and understanding of the classification criteria.
On the other hand, Automated Document Classification uses software tools, algorithms, or machine learning models to automatically categorize documents into predefined classes or categories. Here, documents are analyzed and classified based on their content, textual features, structural elements, or other relevant characteristics using computational techniques. Automated document classification systems can leverage various machine learning algorithms, such as supervised learning, unsupervised learning, or deep learning, to learn patterns and relationships from labeled training data and apply them to classify unseen documents.
While manual classification has been traditionally used for document categorization, the limitations associated with human subjectivity, labor-intensiveness, lack of learning capability, and scalability make automated classification the preferred approach. So, here we will focus more on using automated classification systems in managing and processing document repositories.
The Working of Automated Document Classification
Automated document classification is divided into three essential levels.
Level 1: The first step is to identify and classify the file format. The file format can range from being a jpeg, doc, pdf, mp4, etc.
Level 2: The second step identifies the overall structure of the document, and this is one of the crucial steps of automated document classification.
This is again divided into three separate categories.
1. Completely Structured
The documents in this category utilize a fixed template and they are easy to sort through. The data within these documents may vary but the overall format is always the same. These may include admission forms, Tax returns, or documents with tables such as bank statements.
2. Partially Structured
Now, in these documents, there will be trends of similar templates, but the data will not be completely structured. The data present will be out of place. A perfect example can be a patient’s medical history.
3. Unstructured
As the name suggests, the documents will have no commonalities with each other. There will be no common structure or key value points to sort them. The best example of such documents can be contracts or other legal documents such as divorce agreements.
Level 3: This is the final step that allows the classification of the documents. The documents are sorted into their respective categories by distinguishing the text through techniques such as binarization and noise reduction. These techniques enhance the quality of documents to be processed. To classify the documents, two methods are usually used.
1. Visual Approach
The approach is highly effective with structured documents as the machine does not read the text. Instead, it analyzes the overall structure of the document and identifies the patterns used. The advantage of this method is that it is time-saving but the disadvantage is that it is not very efficient, especially with unstructured documents.
2. Text Classification Approach
The benefit of this approach is that it reads and analyzes the text present in the documents, making it highly effective but also making it a time-consuming process.
Challenges of Traditional Automated Document Classification
Even though automated document classification is a useful technology, it still comes with challenges.
- Dealing with documents that have missing constraints reduces the overall precision of the classification.
- Require a large sample size to train the algorithm.
- Accurately reducing the noise from documents without losing the essential information.
- Classifying jargon used especially in chats, messages, and other human-based informal replies.
- Constantly training the AI algorithm with varying key filters that are found in unstructured documents.
- Dealing with poor-quality scans due to camera quality, weak software, human error, etc.
These challenges may be partially addressed using GPT technology.
Use of GPT for Document Classification
The use of GPT (Generative Pre-trained Transformer) for document classification represents a significant advancement in the field of natural language processing (NLP).
The strength of GPT models in document classification lies in its ability to grasp the subtle nuances and complex patterns within the text, enabling it to make accurate category predictions.
GPT, such as the widely recognized GPT-3 and GPT-4 models, can be fine-tuned on a specific, smaller dataset tailored for the task of classifying documents into predefined categories. This fine-tuning process involves adjusting the model's parameters so that it can better understand and categorize new, unseen documents based on their content.
When fine-tuned for document classification, the model learns to apply the general knowledge to the specific task at hand, effectively distinguishing between different types of documents.
As a result, GPT models can be an invaluable tool for document classification tasks.